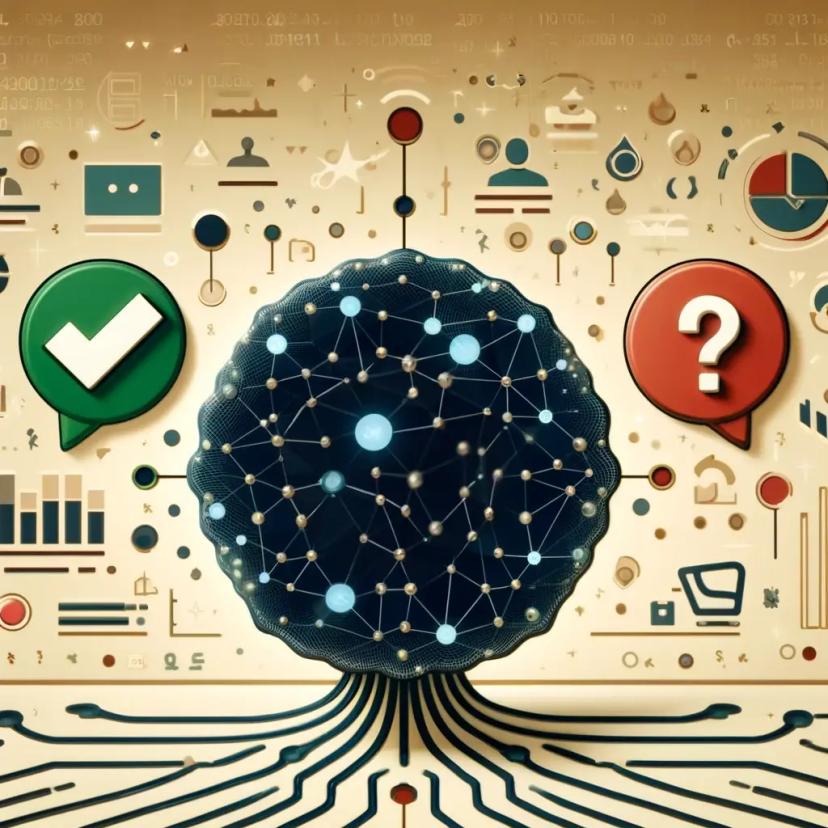
Calibrating Large Language Models Using Their Generations Only
arXiv, 2024As large language models (LLMs) are integrated into user applications, accurately measuring a model's confidence in its predictions is crucial for trust and safety. We introduce APRICOT, a method that trains a separate model to predict an LLM's confidence using only its text input and output. This method is simple, does not require direct access to the LLM, and preserves the original language generation process.